Ultimate Guide: AI vs ML vs Deep Learning vs Generative AI
In today's world where technology is everywhere, the term Artificial Intelligence (AI) gets thrown around a lot. It often gets mixed up with other big words like Machine Learning (ML), Deep Learning (DL), and Generative AI (GenAI). Even though they all sound similar and are related, each one has its own special features and uses. This guide will help clarify what makes each of these technologies unique characteristics and how they're changing our digital world.
ARTIFICIAL INTELLIGENCE (AI)
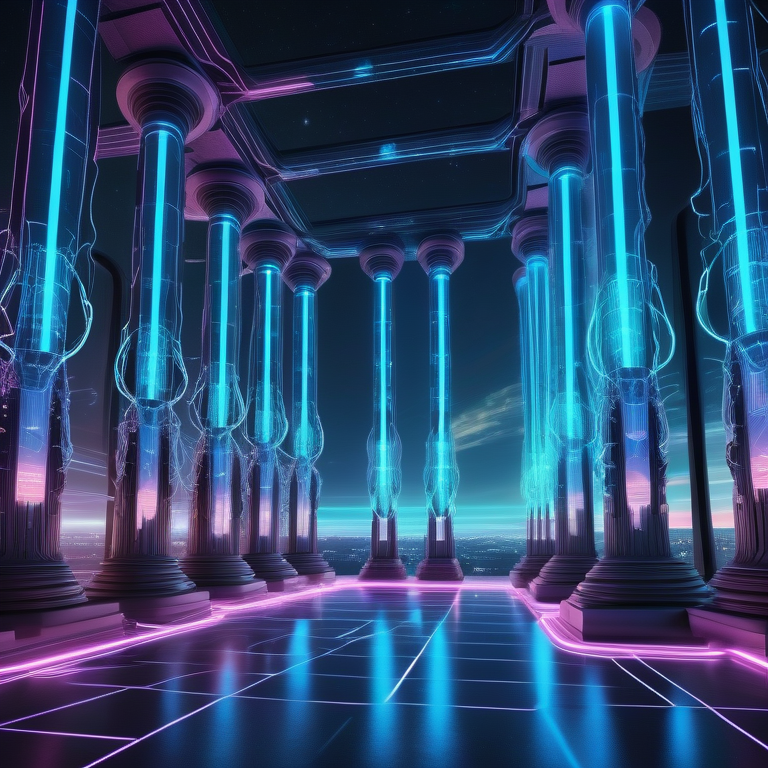
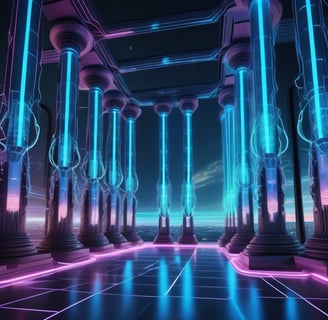
Key Highlights
At the heart of today's computers is artificial intelligence (AI), which lets machines do tasks that normally require human smarts.
With Machine Learning, a part of AI, algorithms get better by learning from data and then can predict or make choices.
Deep Learning pushes what AI can do even further. It uses artificial neural networks to learn deeply and decide on things.
Generative AI is all about creativity in AI. It focuses on making new stuff instead of just looking at what already exists.
Together, AI, Deep Learning, and Generative form a layered set-up contributing to the bigger picture of artificial intelligence technology.
Understanding how these technologies differ helps us see their unique roles in building tomorrow's tech world.
Introduction
In today's world where technology is everywhere, the term Artificial Intelligence (AI) gets thrown around a lot. It often gets mixed up with other big words like Machine Learning (ML), Deep Learning (DL), and Generative AI (GenAI). Even though they all sound similar and are related, each one has its own special features and uses. This guide will help clarify what makes each of these technologies unique characteristics and how they're changing our digital world.
At its core, AI is what powers modern computers to act somewhat like humans. It covers a wide range of methods that allow machines to understand things, make decisions, learn on their own, and even reason out problems. ML falls under the umbrella of AI; it's about giving machines data so they can learn by themselves without someone having to program them for every task. DL goes even further into this idea by using something called artificial neural networks which have different layers for processing information in more complex ways. GenAI takes a piece from DL but focuses specifically on making new stuff that didn't exist before.
By getting the hang of how these technologies differ from one another we can see just how much impact they have across various fields - be it healthcare or fashion design or driving cars without human help! Each plays an important role in pushing forward innovations in their areas by doing things better than before.
Exploring AI, ML, Deep Learning, and Generative AI
Artificial intelligence, or AI for short, is like the big umbrella that covers everything machines can do which normally requires a person's smarts. Under this huge umbrella are things like machine learning (ML), deep learning (DL), and generative AI (GenAI). Machine learning is all about teaching computers to learn on their own by feeding them loads of data so they can make smart guesses or decisions later on. Deep Learning goes even deeper into what ML does by using something called artificial neural networks; think of it as trying to mimic how our human brains work but inside a computer. Then there's Generative AI, which takes things up a notch in DL by focusing on making brand-new stuff that didn't exist before, showcasing the ability of machines to create and innovate.
All these parts - from the broad abilities of machines with artificial intelligence to the specific tasks handled through machine learning, down to creating original content with generative AI using complex models found in deep learning - play different roles in the development of intelligent machines and applications. Together, they push forward what we know and love about technology today.
1. Artificial Intelligence (AI): The Foundation of Modern Computing
Artificial Intelligence, or AI for short, is like the brain behind today's computers. It is a discipline, a branch of computer science, that deals with the creation and development of machines that think and act like humans. This includes a bunch of cool stuff like machine learning, understanding human language (that's natural language processing), robotics, and helping computers "see" things (which we call computer vision). The idea is to make these AI systems smart enough to learn from all the information they get and keep getting better at tackling tough problems over time. They're super useful in lots of areas - think about doctors figuring out what makes us sick or companies figuring out how to move things around more efficiently.
With AI, we're trying to teach machines to understand us when we talk, recognize different objects in pictures just by looking at them, and even make their own decisions based on what they've learned. These intelligent systems are really good at going through tons of info quickly so they can help humans make smarter choices faster than before while also cutting down on mistakes and saving money. But it's not all smooth sailing; there are some tricky questions about whether it’s always okay how these AIs use our personal info or if sometimes they might be making unfair decisions because of hidden biases.
2. Machine Learning (ML): AI's Dynamic Offspring
Machine learning, a branch of AI, is all about creating algorithms and statistical models that learn from data to predict or make decisions. By training these ML algorithms on lots of existing content, they pick up patterns and traits within the data. After this training phase, they're ready to predict outcomes for new stuff they haven't seen before, making the machine learning model a crucial component of AI systems.
With machine learning, there are three big types we talk about: supervised learning where the model learns from examples with known answers; unsupervised learning which deals with figuring things out without any specific guidance because it only has input but no labeled outputs; and reinforcement learning that's kind of like teaching by reward or punishment based on actions taken. Another type of machine learning, supervised learning, is when the model is trained on labeled data and learns to accurately map inputs to outputs. This type of machine learning is commonly used in various industries and is an important aspect of AI.
In terms of what machine learning can do across different fields - think of recognizing speech as in natural language processing, spotting faces in photos for image recognition, sniffing out frauds before they happen, or even tailoring ads just for you. It’s great at sifting through tons of information quickly to find useful bits leading to smarter choices and solving problems better, such as in the case of speech recognition. But it's not perfect – if the info it learns from isn’t good (like biased), results might be off too. Plus getting enough of the right kind of data (labeled ones) can be tough but necessary for those accurate results everyone wants.
3. Deep Learning: Diving Deeper into AI's Capabilities
Deep learning (DL) really takes what AI can do and dives deeper. It is a subset of machine learning that uses something called artificial neural networks that have lots of layers to figure out data in a way that's kind of like how we learn things step by step. These deep neural networks are great at spotting complicated patterns and figuring out relationships in the data, which is super helpful for stuff like recognizing images or speech, understanding language, and even making cars drive themselves.
With DL models, you've got several layers made up of artificial neurons. Starting with the input layer where all the raw data goes in, it then moves through hidden layers where the model learns different features from this data. At the end of it all is an output layer that gives us our final answer or decision based on everything we learned along the way.
This whole deep learning thing has been a game-changer in areas such as computer vision—this means machines can now identify and classify objects almost as well as humans can! It’s also totally changed natural language processing; thanks to DL, machines get better at understanding and creating human-like text or speech. However, these models need tons of data to learn properly and they require heavy-duty computing power too. Plus, they're not so easy to understand when trying to figure out how they make their decisions, especially in the case of language translation.
4. Generative AI: The Creative Frontier of AI
Generative AI, or GenAI for short, is like the creative side of AI that's all about making stuff up from scratch using human intelligence. Instead of just looking at what's already there and trying to figure it out, GenAI goes a step further by whipping up its own images, text tunes, or even whole video clips.
At the heart of GenAI are some cool tricks called generative adversarial networks (GANs) and variational autoencoders (VAEs). With GANs, you've got two parts: one part tries to make new things that look a lot like what it has learned from before; the other part plays judge by trying to spot if these new creations are real or fakes. As they keep going at it, the maker gets really good at fooling the judge with super realistic stuff. On another front VAEs get smart about understanding how all kinds of data should look and then use that knowledge to create more things in line with those patterns.
GenAI isn't just playing around—it’s doing serious work across art music gaming content creation bringing into existence unique pieces never seen before But we can’t ignore this technology comes with big questions especially when people might use it for creating fake news videos known as deep fakes To tackle this developers are working hard on ways to keep Generative Ai in check ensuring its powers used for good not mischief
How AI, ML, Deep Learning, and Generative AI Intersect
AI, ML, DL, and GenAI are all parts of a big family in the tech world. Think of AI as the umbrella term that covers everything. Under this umbrella, ML is like learning from data to make smart decisions or predictions. It's kind of like teaching machines how to learn on their own by feeding them lots of information.
Then we have DL, which is a part of ML but goes even deeper. By using something called deep neural networks—imagine these as complex brain-like structures—it can understand and process data in layers upon layers.
Within DL lies Gen AI, which gets really creative by generating new stuff that hasn't been seen before.
So basically:
ML lays down the basics for machines to learn from past info.
DL dives deeper with its artificial brains made up of many layers to grasp complicated patterns.
And then there's GenAi, taking creativity under its wing within those deep learning processes.
Each one builds on top of the other and plays a unique role in pushing forward what technology can do for us today. By getting how they stack up - sorta like blocks in a tower - it helps us see just how cool and important each block is for creating tomorrow’s tech wonders.
Understanding the Hierarchical Relationship
AI, ML, and DL have a kind of step-by-step relationship where each one is built on the top of the other. Think of AI as an umbrella term that covers everything including ML and DL. Within this big category, ML is all about teaching machines to learn from data using statistical methods. Then we've got DL which falls under ML but goes even deeper by employing deep neural networks to understand complex patterns in data.
At the start of it all stands AI, covering a wide array of techniques and uses. As a subset of AI, ML digs into learning from data with statistics at its core. Following up on that is DL; another layer down that focuses specifically on using deep neural networks for sifting through data in layers - making sense of really complicated stuff! Deep learning algorithms, powered by neural networks, are at the forefront of this field and are constantly evolving thanks to the efforts of teams like the IBM Data and AI Team. Understanding the hierarchical relationship between AI, ML, and deep learning is crucial in grasping the capabilities and potential of these technologies.
By getting how these three are stacked upon each other – like blocks in a tower – we get why they're important individually yet super powerful when combined together! With every level (from AI to ML to DL), what machines can do gets more intricate thanks to those foundational elements below them pushing upwards.
From Data Processing to Decision Making: The Flow of Innovation
In the world of AI, ML, and DL, turning data into smart decisions is a big deal. It all begins with data science - this is where we gather, tidy up, and dig through data to find useful bits of information and patterns. After that comes machine learning (ML) algorithms; they take this cleaned-up data to learn from it and start making guesses or choices about what might happen next. Deep Learning (DL) takes things up a notch by using deep neural networks to get even better at spotting complicated patterns in the data.
These insights and educated guesses made by ML and DL are super helpful when it's time to make decisions. For instance, in places like factories, predictive maintenance models that come from ML and DL help figure out when machines might break down so they can be fixed before causing any trouble. In healthcare settings, recommendation systems can use ML to analyze medical data and provide personalized treatment plans for each patient. These technologies also have the potential to improve user experiences by providing personalized recommendations for products, movies, music, and more based on user preferences and behavior.
So basically, the journey from handling heaps of raw info towards making clever moves showcases how businesses can use AI along with its buddies—ML & DL—to not only decide smarter but also streamline their operations while pushing boundaries further.
Differences: GenAI vs Machine Learning vs Deep Learning vs LLMs
GenAI, ML, DL, and Large Language Models (LLMs) are all important parts of AI but they each do something a bit different. GenAI is really good at making new stuff that hasn't been seen before. With ML, it's like teaching computers to learn from the information they get. Then there's DL which goes even further by using deep neural networks so machines can understand more complicated things. LLMs are pretty cool because after reading lots and lots of text data, they can write stuff that sounds like it was written by a person.
Together these technologies have their own special jobs: GenAI helps in coming up with original content; through ML computers get smarter by learning from data; DL uses deep neural networks to grasp the tricky patterns; and LLMs? They're amazing at creating text that feels real.
Real-World Applications and Impact
AI, ML, DL, and GenAI are making big changes in how companies work and how we live our lives. For example, in healthcare, AI helps make better diagnoses and plans for treatment. With manufacturing, ML is being used to predict when machines need fixing before they break down. DL is changing the game with things like facial recognition and understanding human language better which makes stuff like spotting faces or translating languages way easier. GenAI is shaking up the creative world by helping create new kinds of art, music, and writing.
These technologies are really making a difference everywhere you look. They're making things more accurate and efficient while also improving how decisions are made across different fields of work. This leads to getting more done with less money spent along with giving people cooler experiences using these services or products.
As time goes on these tech wonders will keep evolving bringing even cooler ways they can be applied in various industries from natural language processing that understands us better to predictive maintenance keeping factories running smoother without unexpected hiccups.
Transforming Industries with AI and ML
AI and ML are really changing the game across different fields, making things smarter for businesses and how we all use tech. In healthcare, ML algorithms help spot diseases, plan treatments, and find new medicines. For finance matters, AI systems step in to catch fraud, score credits better, and make smart trading moves.
When it comes to getting from A to B safely and efficiently; the transportation sector is betting big on AI and ML with self-driving cars. Over in manufacturing land; these clever algorithms predict when machines will break down before they actually do which helps keep everything running smoothly without unexpected stops. They also make sure supplies are where they need to be in the supply chain just in time.
Shops online or around the corner are using AI too to figure out what you might like even before you do! This means better suggestions for shoppers; keeping shelves stocked just right; plus top-notch customer service that feels personal because guess what? It kinda is thanks to this tech!
So yeah - whether it's health care fixes or shopping hits – AI systems along with ML algorithms have got a lot covered across various industries helping companies not only stay sharp but get ahead by understanding heaps of data quickly so decisions can be made fast leading us into an exciting future as these tools evolve more accessible day by day.
Breakthroughs in Deep Learning: Case Studies
Deep learning has really changed the game in a lot of areas, making machines smarter and more capable of understanding our world. Let's dive into some examples that show off what deep learning can do:
In the realm of computer vision, deep learning models are doing amazing things like recognizing images and spotting different objects. Take the ImageNet challenge as an example; it's this big contest where folks create models to sort images into lots and lots of categories. Deep learning is usually beating the old-school methods here.
When we talk about natural language processing, which includes stuff like translating languages or figuring out if a piece of text is positive or negative, deep learning has made huge strides too. Google’s Neural Machine Translation system is one cool example because it uses deep learning to translate between languages smoothly.
For autonomous driving, which means cars driving themselves without human help, deep learning is super important too. Big names in tech and car industries such as Tesla, Waymo, and Uber are all using these algorithms to make cars that can see their environment and make decisions on their own.
These stories just go to show how powerful deep-learning technology can be when tackling complex tasks across various fields from natural language processing to image recognition in computer vision projects or even helping self-driving cars understand their surroundings better!
Generative AI: Redefining Creativity and Innovation
Generative AI, or GenAI for short, is changing the game by making it possible for machines to come up with new and original stuff all on their own. Imagine a computer whipping up its own pictures, tunes, writings, and even full-on video snippets that nobody has seen before. This is huge because it means people who make art, music or any kind of content can now dive into areas they've never been able to before, including social media. With the ability to automate content generation for social media platforms, Generative AI is redefining creativity and innovation in the world of social media.
With tools like GANs (that's generative adversarial networks) and variational autoencoders (VAEs), these GenAI systems are creating amazing artwork, composing melodies, and penning down stories just by learning from data they're fed. It's like teaching them what exists out there so they can dream up something totally fresh yet similar in vibe. So instead of just understanding or sorting through data we give them; these machines become our creative buddies—coming up with ideas that might not have crossed human minds. Generative AI models, such as GANs and VAEs, are redefining creativity and innovation by producing unique and original content based on vast datasets. This technology is revolutionizing industries and pushing the boundaries of what is possible with AI and machine learning.
By stepping into this new era where machines get creative too; Generative AI isn't just opening doors to unexplored artistic realms but also sparking innovation across various industries by showing us what’s possible when technology gets imaginative.
Machine Learning, Deep Learning, and LLMs Explained: With Top Use Cases
Machine learning (ML) is a part of AI that's all about creating algorithms and statistical models to learn from data and then use what they've learned to make predictions or decisions. There are three big types of ML: supervised learning, unsupervised learning, and reinforcement learning.
With supervised learning, we teach a model using data where we already know the answers. It’s like giving it both questions and answers so that later on it can predict outcomes for new stuff it hasn't seen before. This approach is super useful for things like figuring out if an email is spam, recognizing who or what is in a picture, or deciding if someone should get credit.
Unsupervised learning doesn’t work with pre-answered questions; instead, the model tries to find patterns all by itself. Think of it as letting the model explore freely without knowing exactly what we're looking for—it might group customers based on their shopping habits or spot something odd happening within your computer network.
Reinforcement Learning teaches models through trial and error using feedback from their actions—kinda like training your pet with treats when they do tricks right but aiming much higher—for example, programming robots, winning at games automatically without human input, or driving cars by themselves!
Deep Learning (DL), which falls under machine learning too, uses layers upon layers in neural networks—think brain-like structures—to chew over large chunks of information. These deep neural networks, also known as deep learning networks, are great because they figure out important features directly from raw info, cutting down heavily on manual effort. They are especially good at tasks such as understanding spoken words, just like humans do, talking back naturally, making sense, and even translating between languages. Not forgetting to spot objects in photos accurately.
Generative AI takes this whole game up another notch focusing more on crafting brand-new content rather than simply digesting existing ones Large Language Models LLMs examples being OpenAI's GPT-3 dazzle us by writing text composing music coding almost indistinguishably from humans They shine brightly when tasked with generating readable engaging texts powering chatbots effortlessly flipping between languages plus stepping into creative domains producing art tunes unlike any other
Challenges and Ethical Considerations
While technologies like AI, ML, DL, and Generative AI bring a lot of good things to the table, they're not without their problems. When we start using these tech tools more and more, we have to think about how they might invade our privacy or make us less safe online. There's also the worry that they could be unfair or take away jobs from people.
When it comes to making and using AI systems in a way that's right and fair for everyone involved is super important. We need to make sure these systems don't pick sides because of biased data or algorithms. It’s crucial that they keep our information safe while being clear about what they do with it. And let’s not forget thinking through how all this affects who gets hired for what job – basically looking at the bigger picture of society.
Finding a middle ground where innovation doesn’t step over ethical lines is key if we want Generative AI and other similar technologies to work well for everybody's sake. This means coming up with rules and guidelines so everything runs smoothly while keeping an eye out for everyone’s rights.
Navigating the Complexities of AI Implementation
Putting generative AI, along with other AI systems into use can get pretty tricky. It's not just about having the right tools; it involves a lot of planning and tackling some tough challenges.
For starters, one big hurdle is making sure you have enough of the right kind of data. These AI models are hungry for lots of good quality, well-organized data to learn from. Getting your hands on this data and setting it up correctly isn't quick or easy.
Then there's the issue that comes with needing people who really know their stuff when it comes to tech skills like coding and understanding statistics, not to mention knowing a bunch about the specific area you're working in. Finding folks with these talents can be quite hard.
On top of all that, fitting these new AI technologies into your current ways of doing things poses its own set of problems. You've got to make everything work together smoothly without causing too much disruption or needing a complete overhaul.
We shouldn't forget about thinking through how using AI affects everyone involved ethically – ensuring fairness, openness, and responsibility in how these systems operate is crucial.
Despite all these bumps in the road though, the advantages - like getting more done faster and more accurately thanks to automation - mean diving into implementing generative AI and other ai systems can definitely pay off if approached thoughtfully.
The Ethical Dilemma: Balancing Innovation with Integrity
As the world of generative AI, machine learning (ML), deep learning (DL), and other AI systems keeps getting better, we're facing a big question about right and wrong. It's really important to make sure that as these technologies get more advanced, they are used in ways that are responsible and fair.
One big worry is how these advancements might affect jobs and our smarts. With AI becoming smarter by the day, there's a fear they could take over roles currently filled by people leading to fewer jobs available. We need to think carefully about how this technology affects society and work hard not to leave anyone out in the cold.
On top of this concern is the issue of bias within AI algorithms. Since AIs learn from data given to them if that data has biases those biases can end up being part of what the AI "thinks" or does which isn't fair at all. So it’s crucial we make sure these systems don’t pick up any unfair biases along their way.
When it comes down to privacy concerns too; since many AIs use lots of personal info for training purposes ensuring everyone’s private stuff stays safe becomes super important.
Lastly but equally essential is keeping integrity intact alongside accountability when developing or using such techs making certain respect towards individual rights & societal benefits aren't overlooked
In wrapping things up addressing ethical issues head-on while trying our best to balance cool innovations with doing what's right ensures technologies like generative ai among others benefit us all without stepping on fundamental values.
The Future of AI, ML, Deep Learning, and Generative AI
Looking ahead, the world of generative AI, along with machine learning (ML), deep learning (DL), and natural language processing is on the brink of some really cool changes. These tech wonders are set to keep growing and changing how we go about our daily lives and work.
For starters, in the realm of AI, there's talk about bringing in even smarter technologies like explainable AI and quantum AI. Explainable AI is all about making it easier for us to understand how these smart systems make decisions. On the other hand, quantum AI dives into using quantum computing to tackle super tough problems that regular computers struggle with.
On top of this, another trend picking up steam is mixing together artificial intelligence with stuff like Internet things (IoT) and blockchain technology. By doing this mash-up, we could see intelligent systems that can gather data as things happen around them; they could automate tasks more smoothly or beef up security measures without breaking a sweat.
As we move forward into the future possibilities involving generative AI, natural language, natural language processing, intelligent systems, and computer vision among others will continue evolving too—finding their way into different areas from healthcare to entertainment across various industries.
All in all, the journey ahead for generative AI, machine learning, deep learning, and everything else under its umbrella looks pretty promising—with new advancements emerging left, right, and center ready to redefine our digital world.
Emerging Trends and Future Directions
The world of generative AI and intelligent systems, including AI, ML (machine learning), and DL (deep learning), is always changing. There are a bunch of new trends and future paths that are really shaping up the field.
For starters, there's this cool development happening with better hardware like GPUs and TPUs. These gadgets help speed up how fast AI models learn and make decisions, making everything run smoother and quicker which means we can do more exciting stuff without waiting forever.
Then there's something called edge computing getting mixed in with AI. By doing this, calculations happen right where all the data is collected instead of sending it far away to process. This cuts down on delay time so things like self-driving cars or medical devices can work faster and safer because they don't have to wait as long to know what to do next.
Looking ahead, we're also going to see improvements in explainable AI. This is pretty important because it helps people understand why an AI model made a certain decision by making its thought process clearer.
On top of all that, expect to see smarter systems that blend generative ai, AI with other techs like IoT (Internet of Things)and blockchain creating some groundbreaking changes across different industries by opening up new possibilities for how things operate.
In summary, the journey into the future for generative AI, intelligent systems, and their buddies—AI, Ml, DL—is filled with lots of promise. These advancements aren't just about pushing tech limits; they're set to completely transform our daily lives at home, in cities, and at work.
Preparing for a Future Shaped by Advanced AI Technologies
With the growth of advanced AI technologies, it's crucial to get ready for both the good stuff and the challenges they'll bring along.
Starting with education and getting more skills, as AI gets bigger in our lives, we're going to need a lot more people who know their way around AI. Putting money into learning about AI is key so that we have folks skilled enough to make the most out of these tech wonders, especially as they become more advanced and capable of analyzing large amounts of data.
On another note, making rules and ethical guidelines is also super important. As AIs start doing things on their own, we've got to make sure they do it in a way that's safe and helps everyone. We should always think about what's right when creating AI systems or putting them out there.
Moreover, being open-minded and always willing to learn new things will help us keep up with how fast AI changes. The world of artificial intelligence keeps moving forward quickly; staying curious and innovative can really pay off.
Gearing up for an era dominated by advanced artificial intelligence technologies like this ensures not only tapping into its vast possibilities but also building a future where everyone benefits.
Conclusion
In the constantly changing world of AI, ML, deep learning, and generative AI, it's really important to get what makes each one special. These technologies are not just changing industries but also how we think about creativity. As we deal with their challenges and think hard about right and wrong uses, it's obvious there are so many chances out there waiting for us. By diving into how these AI breakthroughs work in real life, you can stay on top of things. If you're looking for more details or want to work together in this exciting area, feel free to reach out. Let’s confidently step into a future shaped by advanced AI technologies with an open mind.
Frequently Asked Questions
What is the Difference Between AI and ML?
Artificial intelligence, or AI for short, covers a wide range of methods and uses. Within this big area, there's something called machine learning. It's a part of AI that zeroes in on creating algorithms. These aren't just any algorithms; they're special because they can pick up knowledge from data all by themselves and use what they've learned to predict outcomes or make choices without someone having to guide them every step of the way.
How Does Deep Learning Enhance Machine Learning?
Deep learning is a part of machine learning that uses deep neural networks to understand different levels of data. With deep learning, the models can learn directly from unprocessed data and take on complicated jobs like recognizing images and speech. This approach improves how machine learning works by teaching it to handle complex tasks using layers of information.
Can Generative AI Replace Human Creativity?
Generative AI is really cool because it can help make our creative ideas even better instead of taking over. Even though generative models can come up with new stuff on their own, they don't really get the whole picture or how things feel like we do. We still need human creativity and that gut feeling to create things that truly matter and are original.
What Are the Ethical Implications of Advanced AI Technologies?
With the progress in AI technologies, it's really important to think about what's right and wrong. People are worried about how these advancements might affect their privacy, safety, fairness, and society as a whole. To make sure AI helps rather than harms us, we need to create rules that guide its use in a positive way.