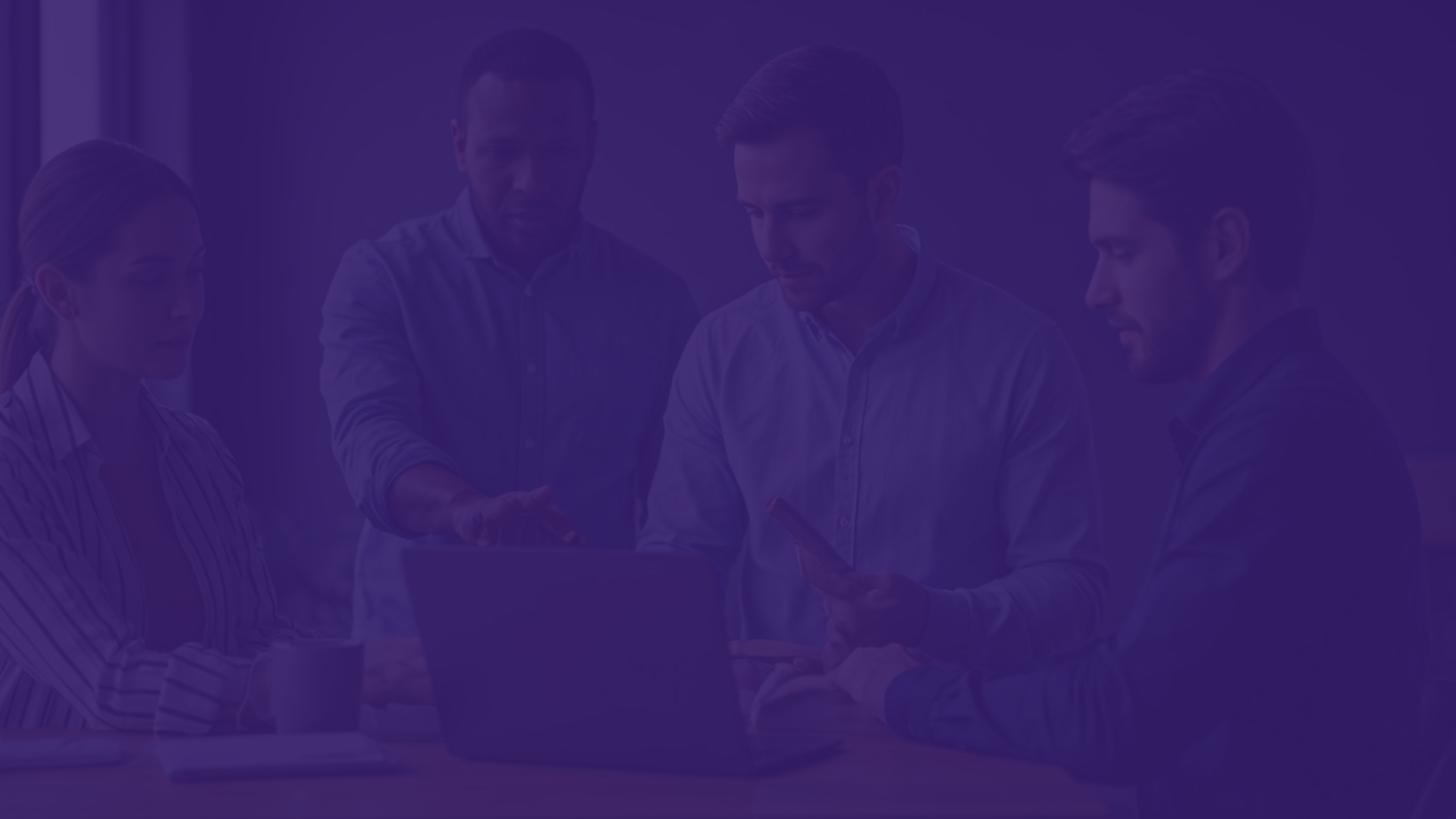
AI in Cybersecurity: How Machine Learning is Shaping the Future of Threat Detection
The field of cybersecurity is always changing. New threats are getting more complicated and harder to handle. Old security measures can't keep up. We need new ideas to keep digital assets safe. AI is changing the future of cybersecurity, especially with machine learning. It helps us find and respond to threats faster and more accurately than before, reducing false positives and improving overall efficiency. This article looks at how machine learning is changing cybersecurity. It will talk about its benefits, the challenges we face, and what the future trends might be in this area.
ARTIFICIAL INTELLIGENCE (AI)
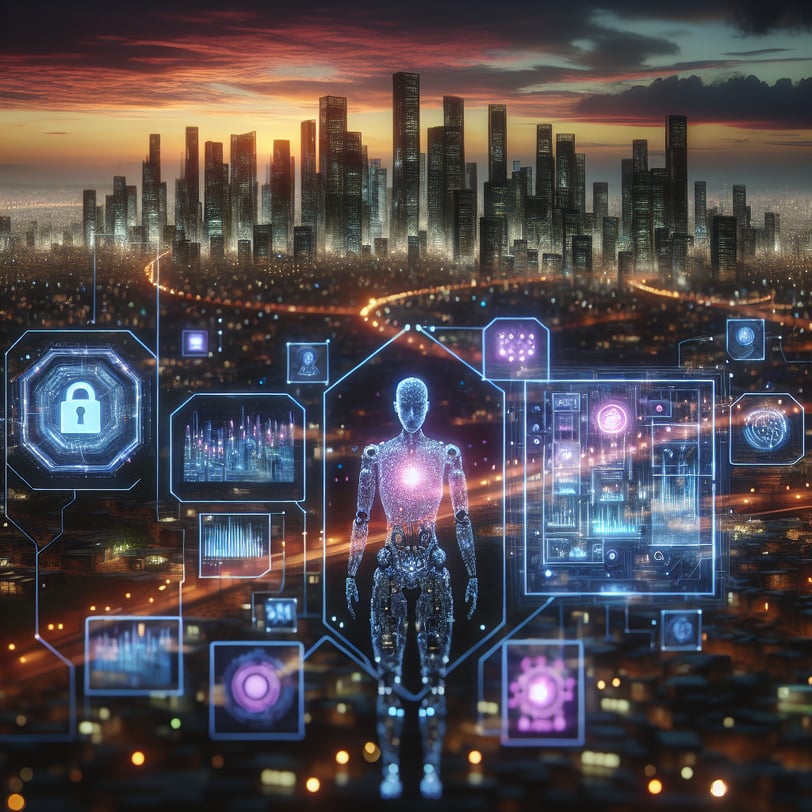
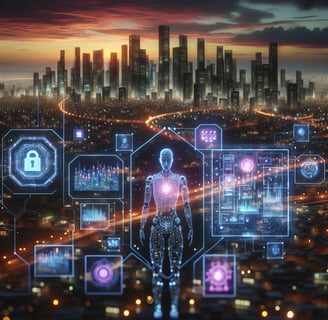
Key Highlights
The increasing sophistication and volume of cyber threats demand innovative solutions, and AI, particularly machine learning, is emerging as a critical tool for strengthening cybersecurity.
AI excels at analyzing massive datasets, identifying patterns and anomalies that often escape human analysts, enabling proactive threat detection and faster incident response.
Machine learning algorithms can be trained on historical data to predict future threats and proactively strengthen security measures.
Despite the numerous benefits, challenges remain in implementing AI in cybersecurity, including managing data bias, ensuring explainability in AI decision-making, and addressing the cybersecurity skills gap.
The future of AI in cybersecurity points towards more autonomous and self-learning systems, deeper integration of deep learning and neural networks, and AI-driven risk prediction.
Introduction
The field of cybersecurity is always changing. New threats are getting more complicated and harder to handle. Old security measures can't keep up. We need new ideas to keep digital assets safe. AI is changing the future of cybersecurity, especially with machine learning. It helps us find and respond to threats faster and more accurately than before, reducing false positives and improving overall efficiency. This article looks at how machine learning is changing cybersecurity. It will talk about its benefits, the challenges we face, and what the future trends might be in this area.
The Role of Machine Learning in Revolutionizing Cybersecurity Threat Detection
The power of machine learning is that it can look at a lot of data and find patterns that people cannot see. This ability can change how we detect threats. Machine learning programs are very good at spotting strange activities in network traffic, user behavior, and system logs. They often give early warnings about possible security problems and take proactive measures to prevent breaches.
Also, these programs learn on their own. This helps cybersecurity systems change and grow as new threats appear. By learning from new data all the time, AI-based security systems can find new threats and attacks easily. They can also guess future weak spots and make security stronger before problems happen.
1. Identifying Patterns and Anomalies in Data for Early Threat Detection
One major benefit of machine learning in cybersecurity is that it can handle and analyze large amounts of data. It finds patterns and unusual signs that may show possible threats. Unlike older systems that rely on fixed rules, machine learning helps cybersecurity systems learn from past data through the use of ML algorithms. This helps them understand what normal behavior looks like.
The ability to detect anomalies is very important for spotting new threats or “zero-day” attacks. These are attacks that do not have specific signatures yet. By noticing strange patterns in network traffic, user actions, or system behavior, smart systems can signal alarms and start security steps. This often happens before any serious damage occurs.
Also, machine learning improves security information and event management (SIEM) systems. It connects data from different sources to find threats that might be missed otherwise. This broad method of threat detection helps organizations strengthen their defenses and respond quickly and well to cyberattacks.
2. Enhancing Phishing Detection with Advanced Machine Learning Algorithms
Phishing attempts are a real danger for both people and organizations. They can often get through standard security measures. Machine learning is a helpful tool to fight against phishing. It looks at things like email content, who sent the email, and website details to spot bad intentions. AI algorithms can learn to see small signs in phishing emails, like:
Suspicious sender addresses and domain names
Misleading subject lines and email content
Malicious links within the email body
By looking at these details, machine learning algorithms can successfully flag potential phishing attacks and keep users safe from falling prey to them. These AI algorithms can also change to meet new phishing methods and get better at finding them over time. This makes them important in the fight against phishing.
3. Utilizing Natural Language Processing to Identify Malicious Intent
Natural language processing (NLP) is a part of AI. It helps machines understand and make sense of human language. In cybersecurity, NLP is important for looking at text data. This data can include emails, social media posts, and online forums. The goal is to find harmful activities.
NLP can understand the meaning and purpose behind what is written. This allows it to spot phishing attempts, social engineering scams, and other online fraud. For example, NLP can find bad links shared on social media. It can also recognize fake messages sent through messaging apps by examining the words and tone used.
As we use more text to communicate online, using NLP to find harmful intent is very important for keeping cybersecurity strong.
4. Improving Malware Detection through Deep Learning Techniques
Traditional ways of finding malware usually depend on signature-based methods. These methods may not work well for new or unknown types of malware. Deep learning, which is a part of machine learning, gives a better solution. It uses artificial neural networks to study malware behavior and spot harmful code.
Deep learning models can learn from large sets of both safe and harmful software. This helps them notice the small differences that make malware unique and separate it from real programs. By recognizing patterns and strange behaviors in code, deep learning algorithms can find malware without needing specific signatures. This makes them good at stopping zero-day attacks and other changing threats.
As malware grows more complex, deep learning techniques offer a strong and flexible way to improve malware detection. This helps lower the chances of security incidents.
5. AI-Driven Behavioral Analytics for Insider Threat Detection
Insider threats can be a big problem for cybersecurity experts. These threats can happen either on purpose or by accident. A strong solution is AI-driven behavioral analytics, specifically anomaly detection. This technology keeps an eye on user behavior and spots any changes from what is normal. These changes might suggest bad actions or hacked accounts.
The system analyzes various activity patterns, using advanced data analysis and machine learning algorithms to detect anomalies in user behavior. This includes things like when users log in, what data they access, and how they communicate through email. Machine learning algorithms help to set a standard for normal behavior for each user. If there are any changes, like trying to access restricted data or strange login attempts, the system flags these as possible insider threats. This leads to alerts for more checking.
With this proactive approach, companies can find and deal with potential risks early. They do this before these issues turn into big security problems.
6. Predictive Analytics in Cybersecurity: Forecasting Future Threats
Predictive analytics uses past data and machine learning to predict future cybersecurity threats and improve security. By looking at patterns in earlier attacks, AI systems can find possible ways hackers might attack and warn organizations about new threats.
A key part of predictive analytics is its power to look at threat intelligence feeds. These feeds share up-to-date information about the latest malware, weaknesses, and attack strategies. By connecting this outside threat data with a company’s current security system, predictive analytics can spot weaknesses and suggest ways to strengthen security.
When organizations can predict and prepare for future threats, they become more proactive in their cybersecurity posture. This helps reduce the damage from potential attacks.
7. Machine Learning in Fraud Detection: Protecting Financial Transactions
The financial services industry uses machine learning a lot for spotting fraud. This technology can look at huge amounts of financial data to find unusual behaviors. Machine learning algorithms can notice what a normal transaction looks like. They quickly mark any transactions that seem different, which could mean fraud.
These models look at many factors, like how much money was involved, where the transaction happened, how often it occurs, and customer habits. This helps them find possible fraud as it happens, stopping losses and keeping customers safe. Additionally, these algorithms can change as fraud tactics change. They keep learning from new data and get better at detecting fraud over time.
Because of this, machine learning is very important for banks and other financial groups. It helps them keep transactions safe and protects customers from losing money.
8. Enhancing Security Incident Response with AI Automation
AI automation is very important in making how organizations respond to security issues better. It helps them deal with cyber threats quickly and correctly. AI-based security systems can look at alerts from different sources, connect data right away, and make incident response tasks easier.
For example, when an AI system sees strange activity, it can stop affected devices, block bad IP addresses, or start response plans without any help from people. This fast response is key to reducing the harm from security breaches and stopping more damage.
By taking care of boring and repetitive tasks, AI allows security teams to focus on the harder parts of responding to incidents. This includes analyzing threats, making plans to fix issues, and reviewing what happened after an incident.
9. The Importance of Continuous Learning Systems in Cybersecurity
Given how fast threats change, learning constantly is key for good cybersecurity practices. Machine learning is very good at this because it can learn from new data and change to match new threats and attack methods.
By always learning from fresh data like threat intelligence, security logs, and malware samples, AI systems can spot new threats that older systems might skip. This ability to change and grow with the threat landscape is vital to stay ahead of cybercriminals and keep a strong security posture.
Organizations that focus on using continuous learning systems in their cybersecurity strategies will be better able to find and handle new threats. This way, they can keep their digital assets safe.
The Intersection of AI and Cybersecurity Ethics: Navigating the Challenges
The advantages of AI in cybersecurity are clear. However, we need to think about the ethical issues that come with using these strong tools in security. AI systems usually learn from large amounts of data. This makes protecting data privacy, dealing with biases, and keeping decision-making clear very important.
It is essential to find a balance between security needs and ethical concerns when using AI in cybersecurity. Organizations should set clear rules about how data is used. They also must address any biases in the training data. Additionally, ensuring human oversight in AI decisions will help build trust and support ethical practices.
Ethical Considerations in the Deployment of AI for Cybersecurity
The use of AI in cybersecurity requires careful thought about ethics. A major worry is the risk of bias in AI algorithms. If the data for these algorithms shows existing biases in society, it can result in unfair treatment of certain people or groups.
For example, if an AI system is trained on data that unfairly points to some demographics as threats, it could cause biased results. This can damage trust in cybersecurity solutions. It is very important to use training data for AI algorithms that is varied, reflect different groups, and is free from bias to stop unfair outcomes.
Transparency and explainability are key to building trust in AI-based cybersecurity tools. Organizations must make sure that AI decisions, like marking suspicious actions or blocking access to certain areas, are clear and easy to understand for users. This clarity supports accountability and helps build trust in using AI for security.
Balancing Privacy with Security: The Role of AI in Sensitive Data Protection
The use of AI in cybersecurity requires a good balance. We need to protect sensitive information while also respecting user privacy. AI systems look at vast amounts of data, which can include personal details, to find security threats.
Organizations must create clear guidelines and strong security measures. This will help keep user data safe and stop unauthorized access or misuse. Tools like data anonymization, differential privacy, and federated learning are important. They allow AI models to learn while still protecting privacy.
It's key to be open with users about how their data is used and protected. Organizations should have clear data usage policies. They need to get informed consent when needed and be honest about their data security practices. This will help build trust in the use of AI for cybersecurity.
Building Trust in AI Systems: Transparency, Accountability, and Governance
Building trust in AI systems for cybersecurity needs a well-rounded approach. This includes being open, responsible, and having strong rules. Organizations must explain how they use AI in their security operations. They should clearly share the goals, advantages, and possible limits of AI with users and stakeholders.
It is important to establish clear accountability for decisions made by AI. This means keeping records of how AI models are created and used. There must be human oversight in important decision-making and ways to fix any mistakes or biases that AI might create.
Having clear rules for AI in cybersecurity is vital for safe use. These rules should cover ethical issues, data privacy, and accountability. They will shape how to develop, apply, and use AI-powered security solutions responsibly.
Overcoming the Barriers to Implementing AI in Cybersecurity
The benefits of AI are making many organizations want to use AI-driven cybersecurity solutions. However, some problems can make it hard to use them well. One big issue is the skills gap. There is a high demand for cybersecurity professionals who understand AI and machine learning.
Another challenge is the complexity of AI systems. These systems need good data for training and decision-making. It’s also important to handle any biases that may come up in AI algorithms. To get past these problems, we need to focus on training initiatives. Collaboration within the cybersecurity community is also vital. Lastly, we must create best practices for using AI responsibly.
Addressing the Cybersecurity Skills Gap with AI Solutions
The rise of AI in cybersecurity has increased the need for skilled workers. These professionals are needed to create, use, and manage these advanced systems. This skills gap in cybersecurity makes it harder for companies to improve their security using AI solutions.
To solve this issue, we need a mixed approach. Schools must create programs that include lessons on AI and machine learning. This will help students gain the skills they need to succeed in this fast-changing field.
Companies should also put money into training programs for their current cybersecurity teams. These programs can give workers the knowledge they need to use AI-powered security tools effectively. By closing the skills gap in cybersecurity, companies can fully benefit from AI to improve their security posture.
Tackling Bias and Ensuring Fairness in AI-powered Security Tools
AI security tools work best when they have good data to learn from. If the data used to train them has biases, the AI models could end up being biased too. This may lead to unfair treatment of certain users. For example, if an AI system wrongly considers some users or their actions as suspicious, it can create biased results, including false negatives.
This is why it's important to fix biases in AI security tools. We need to use training data that is varied, represents different groups, and is free from biases. Doing checks on data quality, using tools that spot biases, and regularly examining training data can reduce the chance of bias.
Also, cybersecurity experts, data scientists, and ethicists need to work together. This teamwork helps create AI security tools that are fair, unbiased, and just. By proactively tackling bias, organizations can build trust in their AI security tools and make sure they are used responsibly.
Managing the Complexity of AI Systems in Cybersecurity Applications
As AI systems get more advanced, using them in cybersecurity can be tough. Setting up, combining, and keeping these systems running needs special skills and resources. Many smaller companies may find it hard to have what they need.
One way to solve this is to team up with cybersecurity vendors that provide AI-powered security as a service. This way, companies can access the needed skills and tools without having to develop and manage them in-house. Still, even when using outside solutions, companies need skilled workers who understand AI. These workers are important to interpret what the systems produce and make sure everything is clear.
People are key to watching over AI systems. They need to check the results and make sure the systems fit with the company’s security plans. Finding the right mix of automation and human oversight is important. This will help manage the complexity of AI systems in cybersecurity and make sure they work well.
Ensuring the Reliability and Resilience of AI Systems Against Adversarial Attacks
AI systems can be very helpful in cybersecurity. However, we need to remember that they can be targeted by attackers. Cybercriminals look for ways to take advantage of weaknesses in AI models. They might change training data, harm algorithms, or try to avoid being detected.
To keep AI systems strong against these attacks, we need a layered approach. Good input validation techniques can help find and deal with harmful data that aims to trick AI models. Adversarial training can also help. This method exposes AI models to tricky examples during training to make them tougher against these kinds of attacks.
It's also important to stay informed about new ways attackers can exploit AI systems. This knowledge helps us create better defenses and keeps AI-powered cybersecurity solutions reliable and effective.
The Future of AI in Cybersecurity: Trends and Predictions
The field of AI is changing quickly. Its role in cybersecurity promises new and smart solutions. Advances in AI areas like deep learning, natural language processing, and computer vision will help improve how we detect and respond to threats.
We can expect to see AI systems that work on their own. These systems can make quick security choices without needing much help from humans. AI-driven cybersecurity will be key in defending against the changing threat landscape. This will help organizations stay one step ahead of cybercriminals and improve their response time to security incidents. It will also help them maintain a strong security posture in the digital age.
Advancements in AI Technologies and Their Implications for Cybersecurity
The fast growth of AI technologies, like natural language processing, deep learning, and reinforcement learning, greatly affects cybersecurity today. As these technologies improve, they give cybersecurity experts better tools to fight new threats.
For instance, advances in NLP help AI systems to look at unstructured data from sources like social media and dark web forums. This helps identify potential threats and malicious activities. Deep learning algorithms are crucial for spotting hidden patterns in large datasets. This leads to better threat detection and prediction of possible future risks.
The mix of AI and cybersecurity is always developing. Ongoing improvements in AI create stronger and more adaptable security solutions for our digital age. Organizations that use these advancements and update their cybersecurity strategies will be better prepared to reduce risks and stay ahead of cyber threats. With the constant evolution of AI technologies, there are endless possibilities for enhancing cyber security and protecting valuable data and systems.
The Evolving Landscape of Cyber Threats and AI’s Role in Defense
The landscape of cyber threats is always changing. Cybercriminals are getting smarter in their methods. Ransomware attacks, phishing scams, and cyber spying by nation-states are big problems for organizations, no matter their size or type.
AI is becoming very important in keeping us safe online. It helps security teams fight these new kinds of threats better. AI systems can look at vast amounts of data from different sources like network traffic, system logs, and threat intelligence. They help spot potential threats and weaknesses in real-time, making AI-powered threat detection systems a crucial tool in defending against cyber attacks.
AI also takes care of basic security tasks. This allows security professionals to concentrate on more complicated threats and make better decisions. Since cyber threats keep changing, we need a proactive approach, and AI is crucial in shaping the future of cybersecurity.
The Integration of AI into Existing Cybersecurity Frameworks and Protocols
Successfully adding AI to current cybersecurity systems is important for organizations to get the most benefits. This process needs careful planning and team effort to make sure that AI tools support and improve current security methods.
A key part of this successful integration is making sure AI solutions match established security rules and routines. This means looking over and updating existing rules to include AI-related issues, like data privacy, reducing bias, and keeping human oversight. Also, combining AI tools with current security information and event management (SIEM) systems can create a central place to watch over and handle security issues.
By smoothly adding AI to current security systems and daily tasks, organizations can use their previous investments while gaining from the extra protection offered by AI solutions. This integration makes sure that AI tools fit well into the organization's overall security posture.
Preparing for the Next Generation of Cyber Threats with AI-Enabled Solutions
The next wave of cyber threats will use advanced artificial intelligence and machine learning more effectively. This means new challenges for security professionals. Cybercriminals may use AI to automate their attacks, create evasive malware, and start tricky disinformation campaigns.
AI solutions are necessary to prepare for and handle these new threats. For example, AI-powered threat intelligence tools can help companies find and study new threats and attack patterns early. Also, AI-based deception technologies can fool and catch attackers, allowing security teams to learn more about their methods.
By using AI, organizations can build a stronger security posture. This will help them be ready for future cyber threats and handle them better. Continuous investment in AI research and development is key to staying on top of the changing threat landscape and keeping digital assets safe.
Conclusion
In conclusion, using AI and machine learning is changing how we protect ourselves from cyber threats. It helps us detect threats better and respond more quickly. New tools like early threat identification, phishing detection, and predictive analytics are making a big difference in the future of cybersecurity. Still, we need to be careful. Ethical concerns, skill shortages, and system issues are challenges we must deal with for these tools to work well. As we face new cyber threats, AI solutions will be key in keeping our systems safe. To stay ahead, it's important to embrace AI in cybersecurity and prepare for upcoming challenges. If you want expert help on how to use AI solutions, get in touch with us.
Frequently Asked Questions
How does machine learning improve threat detection in cybersecurity?
Machine learning improves threat detection. It looks at large amounts of data in real-time. This helps to find patterns and unusual activity. With this, security measures can be taken before problems arise. It also helps in speeding up the response to incidents. Machine learning is good at finding unknown threats. Traditional security methods might overlook them. By using this technology, organizations can enhance their cybersecurity posture.
What are the ethical considerations when using AI in cybersecurity?
Ethical issues arise when using AI for cybersecurity. It is important to use sensitive information responsibly. We must protect user privacy and also work on reducing bias in AI algorithms. Keeping things clear and open about AI-driven security measures is essential. Balancing these factors is key to building trust and encouraging the ethical use of AI.
Can AI replace human cybersecurity experts?
AI is not here to take the place of human cybersecurity experts. Instead, it works alongside them. AI automates repetitive tasks. It also gives insights from large sets of data and helps with continuous monitoring. This teamwork lets human analysts pay attention to more complicated threats and make better decisions.
What are the significant challenges in integrating AI into cybersecurity strategies?
Adding AI to cybersecurity strategies can be tricky. It involves handling the complex nature of AI systems. We need to make sure there is human oversight in decisions made by AI. There are also problems with biases in algorithms to consider. Keeping up with AI technologies that change quickly is another challenge. We need to overcome these issues to successfully adopt AI in cybersecurity.