10 Innovative Applications of Machine Learning in Healthcare
The healthcare industry is changing a lot because of machine learning and artificial intelligence. These tools help medical professionals look at large amounts of patient data points from connected medical devices, enhancing the speed of data collection. They can find important information and make better choices in medical diagnostics. Machine learning is improving how accurate diagnoses are and personalizing treatment plans. This is changing patient care and shaping the future of healthcare.
MACHINE LEARNING (ML)
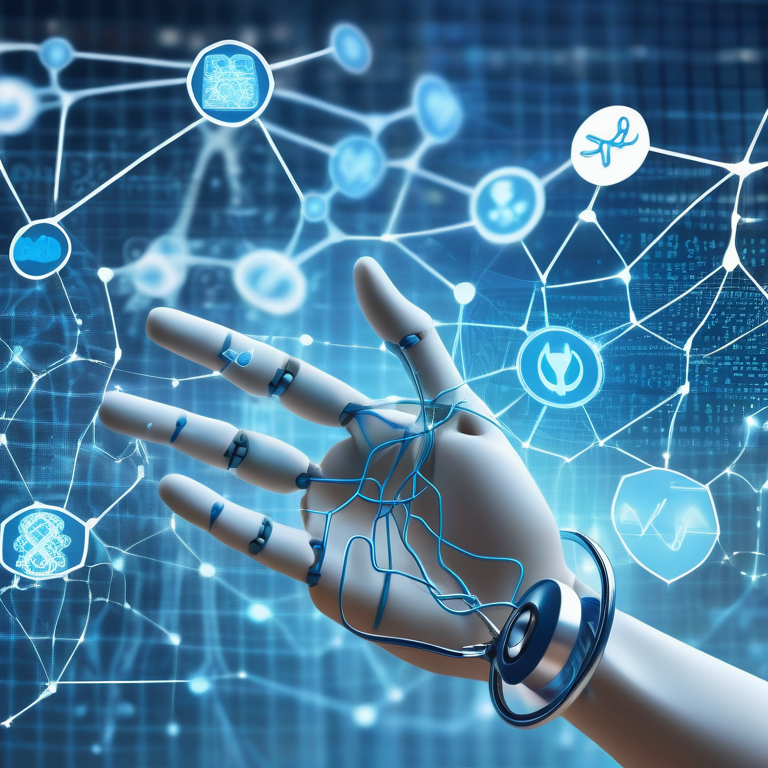
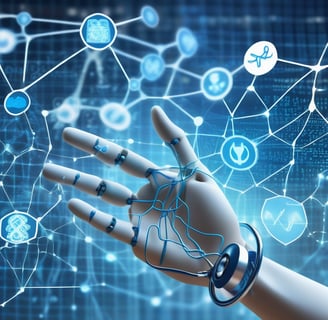
Key Highlights
Machine learning is revolutionizing healthcare with diagnostics, treatment, and research applications.
AI-powered tools enhance diagnostic accuracy, enable personalized medicine, and streamline administrative tasks.
Predictive analytics facilitate early disease detection, improve patient outcomes, and optimize resource allocation.
Robotics and AI are transforming surgical procedures, making them less invasive and more precise.
Ethical considerations and data privacy are paramount in the development and deployment of AI healthcare solutions.
Introduction
The healthcare industry is changing a lot because of machine learning and artificial intelligence. These tools help medical professionals look at large amounts of patient data points from connected medical devices, enhancing the speed of data collection. They can find important information and make better choices in medical diagnostics. Machine learning is improving how accurate diagnoses are and personalizing treatment plans. This is changing patient care and shaping the future of healthcare.
10 Transformative Machine Learning Innovations in Healthcare
As the healthcare industry changes with digital technology, machine learning is becoming an important tool. It helps improve patient care and medical studies. Machine learning can analyze complicated medical data and find patterns. This capability is leading to new ideas that are changing the healthcare field.
This article will look at ten new uses of machine learning in healthcare. It will show how this technology is changing diagnosis and treatment plans, and how well things work.
Predictive Analytics for Early Disease Identification
Predictive analytics uses machine learning to look at patient data. It helps find patterns and predicts the chances of developing certain diseases. By reviewing past medical records, lifestyle choices, and genetic information, these tools can spot people at risk for issues like diabetes, heart disease, or cancer.
Early detection through predictive analytics allows healthcare professionals to act early. They can suggest personal prevention plans and may change how a disease develops. By knowing which patients are at high risk, healthcare providers can improve screenings, start help on time, and get better patient outcomes.
This advanced approach improves patient care. It also eases the strain on healthcare systems by lowering the need for expensive treatments later on.
Enhancing Diagnostic Accuracy with AI Imaging Tools
Artificial intelligence, or AI, is changing the way we analyze medical images with various machine learning (ML) applications contributing to advancements in the field, including image recognition through neural networks. Deep learning algorithms help to improve the accuracy of diagnoses. AI also aids radiologists when they look at complex medical images. AI tools can check images from CT scans, MRIs, X-rays, and ultrasounds. They can find small problems that people may miss.
By automating image analysis, AI helps with:
Finding tumors, fractures, and other issues.
Measuring the size and shape of organs.
Spotting early signs of diseases.
With AI in medical imaging, we have fewer diagnostic mistakes. It makes the reading of images faster and helps provide better patient care.
Machine Learning in Personalized Medicine
Personalized medicine is a major change in healthcare. It aims to create treatment plans that fit each person’s unique genes, lifestyle, and environment. Machine learning algorithms are very important. They help analyze patient data to find the best treatment methods and forecast how each person will respond to therapies.
By looking at a patient’s unique traits, machine learning can help healthcare professionals with:
Choosing the best medication dosage.
Predicting how successful the treatment will be.
Spotting possible side effects.
This way of using data in patient care improves treatment results, reduces negative reactions, and increases patient satisfaction.
AI-Driven Patient Monitoring Systems
AI-powered systems for monitoring patients are changing how healthcare works. They allow for continuous, remote tracking of a patient’s health. Wearable devices, remote sensors, and mobile apps gather real-time health data. This includes heart rate, blood pressure, sleep patterns, and activity levels.
Machine learning algorithms look at this data to find problems, track how diseases change, and notify healthcare providers about possible issues. The ability to check on patients remotely offers:
Proactive interventions.
Early detection of complications.
Reduced hospital readmissions.
These systems give patients more control over their health. At the same time, they give healthcare providers important insights to make better decisions.
Robotics and AI in Surgical Procedures
Robotic surgery helped by artificial intelligence is changing how surgeries are done. It is making them more accurate, less invasive, and safer for patients. Surgical robots, operated by skilled surgeons, provide better control, 3D imaging, and filter out hand tremors. This allows surgeons to tackle complex operations with more precision.
AI algorithms make robotic surgery even better by:
Analyzing surgical images in real-time.
Giving surgeons decision support during surgeries.
Taking care of repetitive tasks.
Combining robotics and AI in the operating room is improving surgical results, cutting down recovery times, and changing the future of surgery.
Virtual Health Assistants for Improved Patient Engagement
Virtual health assistants are changing the way patients work with healthcare services. These smart helpers use artificial intelligence and natural language processing. They can:
Schedule appointments.
Give medication reminders.
Answer common questions.
By handling routine tasks and being available anytime, these assistants help patients stay involved and make it easier to get care. They also lighten the load on healthcare providers. Furthermore, they provide personalized health info, help manage long-term health issues, and encourage following treatment plans.
Machine Learning for Epidemic Outbreak Prediction
Predictive analytics uses machine learning to help with public health and predict disease outbreaks. It looks at big sets of data like health records, travel habits, social media trends, and environmental factors. These techniques can spot potential disease outbreaks early on.
Finding outbreaks early helps public health officials to:
Act quickly with interventions.
Use resources in a smart way.
Slow down the spread of diseases.
AI in Drug Discovery and Development
Drug discovery and development take a lot of time and money. Machine learning techniques are changing this field. They speed up finding new drug candidates, guessing how well they will work, and improving clinical trial designs.
By looking at large sets of data about molecular structures, biological activity, and patient details, machine learning algorithms help:
Find good drug targets.
Guess how drugs will interact with targets.
Improve drug efficacy and safety.
Real-Time Health Data Analysis for Informed Decision Making
The growing access to real-time health data from wearable devices, health records, and other sources is opening up new chances for smart choices in healthcare. Machine learning can study this data as it comes in. This helps healthcare professionals get useful insights right away.
Real-time data analysis helps with:
Early detection of patient issues.
Personalized treatment changes.
Better use of resources.
Machine Learning for Efficient Healthcare Administration
Administrative work takes up a lot of time and resources in healthcare. Machine learning can make these tasks easier. It helps with appointment scheduling, billing, processing claims, and managing inventory.
By automating routine tasks, machine learning makes operations more efficient, lowers costs, and allows healthcare professionals to spend more time on patient care. It also helps with resource allocation, staffing choices, and bed management. This leads to better patient flow and shorter wait times.
The Impact of Machine Learning on Patient Care
Machine learning is changing patient care. It helps medical professionals use data to get better insights. This leads to more accurate diagnoses and personalized treatment plans. With AI tools in healthcare systems, patients get clearer diagnoses, custom therapies, and better health results.
Machine learning also promotes proactive and preventive care. This change is improving how chronic diseases are treated. It reduces hospital readmissions and boosts overall patient well-being.
Elevating Patient Satisfaction through Personalized Care Plans
Personalized care plans are changing the patient experience for the better by integrating unified patient records and utilizing clinical decision-support tools. These plans fit each patient's unique needs and preferences. They lead to higher satisfaction rates. Machine learning helps healthcare professionals by looking at patient data. This allows them to create customized treatment strategies and predict how individuals will respond to therapies. It also helps them find and fix potential issues early.
Personalized care encourages patient engagement. It gives people a role in managing their health and builds stronger relationships between patients and providers. By making treatment plans specific to each person, healthcare providers can see better medicine adherence. This also helps improve treatment outcomes and overall patient satisfaction.
Reducing Hospital Readmission Rates with Predictive Algorithms
Hospital readmissions put a heavy strain on healthcare facilities and systems. They can also show problems in patient care. Predictive algorithms, which use machine learning for anomaly detection, help spot patients at high risk of disease diagnosis in the early stages and returning to the hospital. This way, healthcare providers can step in quickly to avoid serious issues and limit unnecessary hospital visits.
These algorithms look at patient data. They assess factors like medical history, how well patients follow their medication plans, and social details about their lives. By doing this, they can find those who may need extra help after they leave the hospital. This forward-thinking method lets healthcare providers create tailored care plans for patients. They can then link patients to the resources they need. In the end, this helps lower hospital readmission rates.
Advancements in AI-Driven Diagnostics
AI-powered tools are changing how we find and treat diseases. Machine learning makes medical image analysis more accurate and efficient. This helps doctors to detect various conditions, like cancer and heart diseases, sooner and with more precision.
These improvements allow for quicker action, more tailored treatment plans, and better patient outcomes.
Breakthroughs in Cancer Detection Using Machine Learning
Early detection is crucial for successful cancer treatment. Machine learning applications are making significant strides in improving cancer detection rates and accuracy across various cancer types. AI-powered tools are assisting in:
Analyzing medical images to identify suspicious lesions.
Developing personalized risk assessment models.
Predicting cancer recurrence.
Revolutionizing Cardiac Care with AI Algorithms
Heart disease is still a top cause of death around the world. AI systems are changing how we care for hearts. They help doctors in finding, treating, and managing many heart problems. By studying electrocardiograms (ECGs), echocardiograms, and other heart imaging data, AI can do the following:
Find irregular heart rhythms.
Predict heart attack risks.
Create personalized treatment plans for heart failure patients.
These AI tools help cardiologists make better decisions. They can act quickly and improve patient health in heart care.
The Role of Machine Learning in Managing Chronic Diseases
Chronic diseases like diabetes, heart disease, and breathing problems need constant care and personal attention. Machine learning is becoming very important for helping patients and healthcare providers manage these diseases better.
By looking at patient data, tools powered by AI show how diseases are changing, if patients are taking their medicine, and what problems might come up. This helps make quick decisions and tailor treatments to each person.
Diabetes Management Through Predictive Analytics
Diabetes impacts many people around the world. It needs careful checking and tailored management. Machine learning, through predictive analytics, is changing diabetes care by offering:
Personal insights about blood sugar trends.
Quick alerts for possible low or high blood sugar levels.
Suggestions for changing medications and lifestyle choices.
These smart tools help people with diabetes manage their health better. They can make informed choices about their care, which can lead to a better quality of life.
AI-Powered Tools for Continuous Monitoring of Chronic Conditions
Keeping a close watch on chronic conditions is very important. It helps find problems early and get treatment when it's needed. AI tools, like wearable sensors, remote patient devices, and smart inhalers, help gather and analyze information all the time to:
Track how the disease is changing.
Spot early signs of worsening.
Warn healthcare providers about possible problems.
These tools help both patients and healthcare providers to take charge of chronic conditions. They help avoid hospital stays and lead to better health over time.
Ethical Considerations in AI Healthcare Applications
As artificial intelligence is used more in healthcare, we need to think about ethics. This way, we can use advanced technology responsibly and fairly. Important areas to consider include data privacy, bias in algorithms, and openness.
Healthcare groups should focus on keeping patient information safe and private. They should also make sure that AI algorithms are created and used in a fair way without bias.
Ensuring Data Privacy and Security in AI Implementations
Data privacy and security are very important in healthcare. This is true especially because AI often deals with sensitive patient data. Healthcare organizations need to follow strict rules for protecting data, like HIPAA. They also must use strong security measures to keep patient data safe.
AI should focus on anonymizing and de-identifying data. This protects patient privacy while allowing data to be used for research and development. It's also important to have clear policies about data management and to make sure there is clear consent. This helps build trust and ensures ethical practices in AI healthcare applications.
Addressing Bias and Fairness in Machine Learning Models
Machine learning models can have biases from the data used to train them. If healthcare data shows past inequalities or problems in getting care, it may lead to unfair or incorrect predictions. This can make existing healthcare issues even worse.
To fix bias in machine learning models, we need to choose data carefully. We also need to be clear about how our algorithms work and keep checking for any unexpected bias. Using diverse and representative datasets is very important. Regular audits, methods to reduce bias, and ongoing checks can help make sure that AI in healthcare is fair and balanced.
Overcoming Challenges in Integrating AI into Healthcare
The potential of AI in healthcare is very large. However, combining these technologies with current healthcare systems is not easy. There are rules to follow, systems that need to work together, and workers who need training. It takes teamwork from everyone in healthcare to solve these problems.
By addressing these challenges, we can fully use AI in healthcare. This will help ensure that these new technologies are safe, effective, and fair for everyone.
Navigating Regulatory Hurdles for AI Healthcare Solutions
The fast-changing world of AI technology creates challenges for the groups making sure AI in healthcare is safe and works well. Old rules may not fit well with the special features of AI systems. These systems can learn and improve over time.
To manage these rules better, AI developers, healthcare providers, and regulatory agencies need to work together. They should create clear rules for checking AI systems, keeping information open, and being responsible. Flexible rules that move along with technology are very important. This will help keep patients safe and support new ideas in the healthcare sector.
Bridging the Gap Between AI Technology and Clinical Practice
Integrating AI technology into healthcare needs to connect new technology with the real needs of healthcare professionals. Many clinicians may not have the training or understanding of AI to use and understand AI-generated insights well.
To fix this issue, we should include AI education in medical training. We also need to create easy-to-use AI tools that fit into clinical work. Moreover, it is important for healthcare professionals to work together with data scientists. Giving clinicians the right knowledge and tools to use AI technology effectively is crucial for its successful use and acceptance.
Future Directions of Machine Learning in Healthcare
The future of machine learning in healthcare is very exciting. It can change many parts of patient care, medical research, and public health. With AI joining forces with new technologies like genomics and wearable health sensors, we will see new ways to provide personalized and proactive healthcare.
As AI algorithms get better and datasets get bigger, we will likely see even more amazing applications of machine learning in healthcare. This can lead us to a future where technology helps people live healthier lives.
The Potential for AI to Enhance Telemedicine Services
Telemedicine services have grown quickly in recent years. They are set to improve even more with the use of AI. AI tools can help with:
Remote patient monitoring.
Automated diagnosis and triage.
Personalized health recommendations.
By using AI, telemedicine platforms can offer better healthcare services to patients who live far away or have trouble moving around. This use of AI can help connect people with different types of care, make it easier for them to get the help they need, and improve their overall experience.
Innovations in Wearable Health Technology Powered by AI
Wearable health technology like smartwatches, fitness trackers, and biosensors is collecting a lot of information about our health. AI algorithms are very important because they help process this information. They can give personalized advice, notice unusual patterns, and foresee possible health issues.
As we improve wearable technology, AI will lead to new ideas in patient monitoring, managing diseases, and preventing health problems. With AI analyzing health data in real-time, we can take action quickly, get custom health recommendations, and manage ongoing health issues better.
The Integration of Genomics and Machine Learning for Advanced Treatments
Genomics is the study of our genes and how they interact. It has great potential to help us understand and treat diseases. When we combine genomics and machine learning, we can create better treatments, personalized medicine, and more accurate diagnoses.
Machine learning can look at large sets of genomic data and clinical data, playing a key role in the drug discovery process alongside pharmaceutical companies. It helps find genetic markers linked to diseases and identify potential trial participants. It can also predict how people will respond to treatments and create focused therapies. This teamwork between genomics and AI is changing healthcare. It leads to better treatments, earlier diagnoses, and a deeper understanding of the genes behind diseases.
Conclusion
In healthcare, using machine learning is changing how we care for patients. It helps with predicting diseases early. It also makes diagnostics more accurate and creates personalized treatment plans. These changes improve patient satisfaction because care is better designed for their needs. They also help lower the chances of patients returning to the hospital. As we deal with ethical issues and work through challenges, machine learning has a bright future in healthcare. It boosts telemedicine, supports wearable health technology, and integrates genomics. This means healthcare is getting better, leading to advanced treatments and better results for patients.
Frequently Asked Questions
How Can Machine Learning Improve Diagnosis in Healthcare?
Machine learning looks at medical data, such as patient history and medical images. It helps find patterns and spot problems. This support helps healthcare professionals make diagnoses quicker and more accurately.
What Are the Ethical Implications of Using AI in Healthcare?
The ethical issues of AI in healthcare are important. It is vital to keep data private. We also need to look at bias in algorithms. Furthermore, we must be open about how decisions are made.
Can Machine Learning Algorithms Predict Epidemic Outbreaks?
Yes, machine learning can use predictive analytics and pattern recognition to look at health data. By doing this, it can find possible outbreaks of epidemics.
What Is the Future of Machine Learning in Personalized Medicine?
Machine learning will change medicine in a big way. It will create treatment plans that fit each patient’s data. This will lead to new ideas in healthcare that focus on what each person needs.